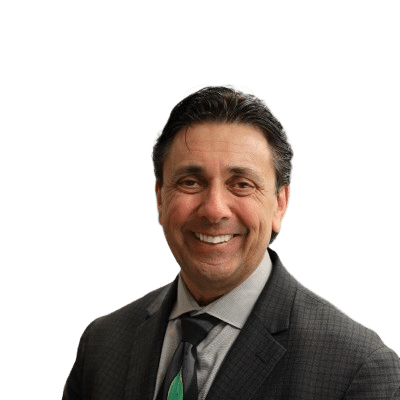
Director of Innovation in AI and Analytics
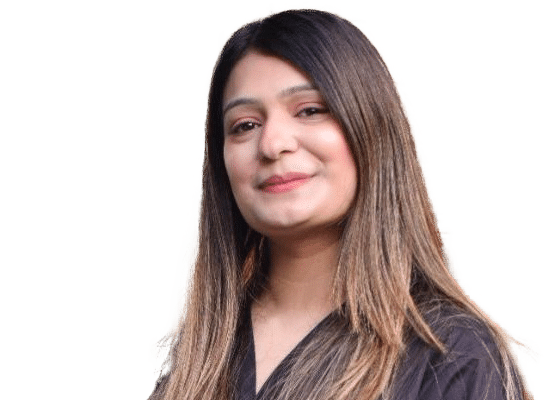
Data & AI Evangelist
Subscribe to the newsletter
As businesses grapple with ever-increasing data volume, velocity, and variety, the need for robust solutions for storing, managing, and analyzing data becomes paramount. A survey reveals that a quarter of decision-makers believe their companies’ data volumes will expand by over 60% by the end of 2024. This emphasizes the need for scalable data storage solutions to accommodate this rapid growth.
Two prominent data management solutions that effectively address this challenge are data Lakehouse and data warehouse which have emerged as strong contenders in the data storage and analytics market. Both these data repositories assist businesses in managing and analyzing their big data, creating insights, and jumping to conclusions that shape business decisions.
However, the question arises: if both are prominent central repositories for storing large volumes of data, why do organizations debate between using a data Lakehouse and a data warehouse for data storage and analytics.
Let’s explore the answer to this question by touching upon the comparison of key features between Data Lakehouse Vs Data Warehouse through this difference guide.
What is a data management solution?
A data management solution refers to tools, processes, and strategies designed to efficiently collect, store, organize, and analyze data. It encompasses various aspects of handling data throughout its lifecycle, including storage, integration, governance, security, and accessibility.
Data management solutions aim to ensure that data is accurate, secure, and readily available for use by organizations to support decision-making, operational efficiency, and business intelligence initiatives. These solutions include technologies such as databases, data warehouses, data lakes, data governance tools, and data integration platforms, among others.
What is a data warehouse?
A data warehouse is a storage solution for storing structured data from multiple sources. This centralized repository of business data containing structured data represents a single source of “data truth” and helps organizations with business intelligence reporting. Businesses use structured data for reporting and visualization purposes. Data warehouses provide data in a row-based or columnar format, simplifying user interaction and enabling faster actionable insights for decision-making within the organization.
A data warehouse is often used interchangeably with a data lake, which is also central to data management solutions. However, there is a significant difference between the two. A data warehouse stores structured, processed data optimized for fast queries and business reporting, while a data lake holds raw, unprocessed data in various formats, offering flexibility for future analysis — making the distinction between data lake vs data warehouse important for modern data strategies.
Data integration in data warehousing: How ETL ensures quality and consistency
Before the data gets stored in a data warehouse, it undergoes a comprehensive process called data integration that collects and prepares data for storage. Data warehouse uses the ETL approach to transform data to ensure quality and consistency. The ETL process works by:
- Extracting data from multiple sources
- Transforming, cleaning, and converting the data into the desired format
- Loading the data into the data warehouse
This process is a key part of the data warehouse architecture, enabling data to be collected, stored, processed, and accessed efficiently. A well-defined architecture ensures that once data is loaded into the warehouse, it can be seamlessly queried and analyzed, providing reliable insights for decision-making. This architecture supports the smooth flow of data from integration to end-user access.
Read more: Understand the concept and process of ETL approach in detail.
Cloud-based data warehouses are another scalable, flexible solution offering safe and secure data storage, processing, integration, cleansing, and loading of data within a public cloud environment. Some of the most popular data warehouse tools are Snowflake, Google Big Query, Amazon Redshift, and Azure SQL Data Warehouse. These data warehouse solutions function as a unified repository for data integrated from various sources to provide meaningful business insights for decision-making.
Traditional data warehouse vs cloud data warehouse: Understand your data storage needs
Unlike traditional data warehouses that offer on-premises storage, cloud data warehouses provide scalable storage in the cloud. This means you can easily adjust storage capacity based on your data needs without the burden of managing physical hardware and infrastructure. This flexibility and cost-effectiveness are major advantages of cloud data warehouses for businesses of all sizes.
What is Data Lakehouse?
A Data Lakehouse is a modern data architecture that combines the features of a traditional data warehouse and data lake. They can support all types of data (structured, semi-structured, and unstructured) and enable cutting-edge business intelligence and machine learning capabilities.
Data Lakehouse architecture addresses the concerns of data scientists, data engineers, and traditional data warehouse professionals for business intelligence and reporting. They handle raw and structured data and use ELT processes to transform and load data to make it ready for reporting and analytical querying. Data Lakehouse supports advanced querying with SQL, making them compatible with a range of analytics tools and frameworks for historical analysis.
Databricks, Amazon Redshift Spectrum, and Google Big Query are some data Lakehouse examples that allow organizations to store and access big data quickly and more efficiently.
A quick comparison of data lake vs data warehouse vs data Lakehouse
A Data Lake is a highly flexible solution that supports both structured and unstructured data in its raw and original format. Unlike a data warehouse that only stores “structured data”, a data lake allows storing all data types for data analysis. Also, a data lake offers flexibility and durability to derive advanced insights from unstructured data. In contrast, data warehouses face challenges when handling this type of data.
The Data Lakehouse approach brings together the features of both data lake and data warehouse, offering analytics flexibility with diverse data types. The table below summarizes the differences between data warehouse vs data lake vs data Lakehouse.
Feature | Data Lake | Data Warehouse | Data Lakehouse |
Storage data type | Structured, semi-structured, and unstructured | Structured | Structured, semi-structured, and unstructured |
Purpose | Store raw data for data exploration and analysis | BI reporting and structured data analysis | Unified data storage and processing for BI and AI/ML |
Cost | Low-cost storage, pay-per-use | Higher cost due to infrastructure and maintenance | Moderate cost with efficient storage and compute |
Schema | Schema-on-read | Schema-on-write | Schema-on-read and schema-on-write |
ACID compliance | Not typically ACID compliant | Fully ACID compliant | Supports ACID compliance for transactions |
Read more: Explore the detailed differences between data lake and warehouse here.
Microsoft’s innovative data management solutions for modern businesses
Since making sense of a large pool of data sets presents a substantial challenge to businesses of all sizes, Microsoft came forward with its innovative offerings to cater to the data management challenges. Microsoft, a leader in cloud computing, offers innovative solutions like Microsoft Fabric and Azure to meet the needs of different organizations regarding data storage and management. These platforms cater to various data storage needs, providing data Lakehouse and data warehouse options.
Understanding the differences between Fabric Lakehouse vs. Warehouse and Azure Data Lakehouse vs. Warehouse allows organizations to choose the right solution for their data needs, whether the focus is on data exploration and advanced analytics, or business intelligence and reporting.
Microsoft Fabric’s contribution
Microsoft Fabric has emerged as a clear leader in managing a diverse pool of data with its warehousing and Lakehouse capabilities. These allow data professionals to efficiently process, analyze, and derive actionable insights from diverse datasets. Let’s explore the capabilities of Lakehouse and warehouse in Microsoft Fabric.
Microsoft Fabric | Warehouse | Lakehouse |
Data volume | unlimited | Unlimited |
Type of data | structured | Unstructured, semi-structured, structured |
Data organized by | Database, schemas, tables | Folders and files, databases, and tables |
Multi-table transaction | Yes | No |
Primary developer skill set | SQL | Spark |
Microsoft Azure’s contribution
Microsoft Azure has also been one of the reasons behind the success of data-driven organizations due to its efficient storage solutions. It provides them with scalable storage solutions to help them keep pace with the ever-increasing amount of data they generate. The table below highlights the difference between a data Lakehouse and a data warehouse in Azure.
Azure | Warehouse | Lakehouse |
Data type | Primarily structured data | Unstructured, semi-structured, structured |
Data storage | Uses SQL-based storage (e.g., Azure Synapse Analytics) | Uses a combination of SQL-based storage and data lake storage (e.g., Delta Lake) |
Integration | Integrates with various Azure services (e.g., Power BI, Azure Machine Learning) | Integration with Azure services, plus support for open-source frameworks (e.g., Apache Spark) |
Performance | Optimized for high-performance queries on structured data | High-performance queries with ACID transactions on various data types |
Purpose | Business intelligence, reporting, and analytics | Data exploration, advanced analytics, unified data management |
Data Lakehouse vs data warehouse: Which data storage architecture is ideal for your data needs?
It is important to understand that “one-size fits all” concept isn’t applicable when it comes to data warehouse vs data Lakehouse. The preferred option depends on the need and the workflow of your organization. However, we have prepared a general guideline to help you choose the right option for your organization’s data storage needs on the go.
- When to use a data warehouse?
To analyze structured and historical data for business intelligence and reporting purposes.
- When to use a data Lake?
For storing, processing, and securing large amounts of structured, semi-structured, and unstructured data for streaming, machine learning, and data science scenarios.
- When to use a data Lakehouse?
To centralize multiple data sources and simplify data engineering challenges by making data available to everyone – democratization.
Data storage and management continue to evolve rapidly, offering diverse architectures like data warehouses and data Lakehouse to cater to varying organizational needs. While data warehouses excel in structured data analytics and established BI practices, data Lakehouse provides a unified platform for handling structured, semi-structured, and unstructured data with enhanced scalability and flexibility.
However, making the choice for the right architecture depends on factors such as data complexity, analytics requirements, and scalability goals. As organizations identify their data storage and management strategies, understanding these differences and aligning them with business objectives will be valuable in making the most out of their data assets.
Transform your data analytics with tailored Data Management solutions
Whether you choose a data Lakehouse or a data warehouse for your storage needs, these data architectures support and enhance your organization’s data analytics capabilities. To optimize your data management and get the most out of your analytics, consider Confiz’s comprehensive data management services. Our team of experts can help you implement and maintain the ideal data architecture tailored to your specific needs. We offer data management solutions that optimize data workflows, improve decision-making processes, and foster innovation within your organization.
Contact us today at marketing@confiz.com to learn more about how we can support your data journey and drive actionable insights for your business.