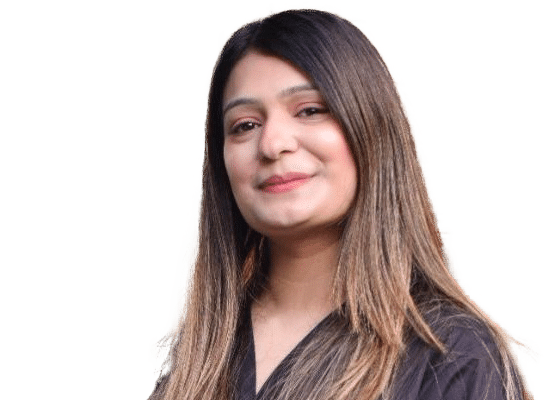
Data & AI Evangelist
Subscribe to the newsletter
Generative AI has rapidly ascended the priority list for executives, with C-suite leaders racing to understand its transformative potential and integrate it into their digital strategy. While CEOs are envisioning a future transformed by AI, CIOs are dealing with the complexities of deploying generative AI at scale. Every day, generative AI news highlights groundbreaking advancements, and for good reason – McKinsey projects that Gen AI’s impact on productivity can add trillions of dollars in value to the global economy.
While the potential is immense, there’s a crucial reality check. Unlike previous technology shifts, generative AI isn’t just another technology rollout, it’s a fundamental change in how enterprises interact with data, make decisions, and even build products.
While successful adoption requires collaboration across multiple stakeholders – CTOs, data scientists, legal teams, and business leaders – the CIO holds the greatest responsibility when it comes to generative AI adoption. As the primary architect of an organization’s digital strategy, the CIO must navigate technical complexities, ensure compliance, manage risks, and align AI initiatives with business goals. Their leadership is essential in driving enterprise-wide AI adoption that is both scalable and responsible.
So, what must CIOs absolutely get right before deploying generative AI solutions?
This blog unpacks the critical factors CIOs must consider before diving into generative AI development. From data governance to ethical AI usage, we’ll explore how organizations can move beyond the hype and implement Gen AI in a way that drives real business value. Let’s break down what it takes to make generative AI a strategic asset, not a challenge.
No 1: Align AI initiatives with core business objectives
Before getting started with your generative AI journey, CIOs must take a step back and ask: What are we actually trying to achieve? The goal isn’t just to have AI, it’s to use it in ways that drive meaningful results, whether it’s about enhancing customer satisfaction, streamlining operations, or unlocking new revenue streams. The key is to start with specific, measurable outcomes. Are you rolling out AI-powered chatbots to cut response times and boost customer engagement? Do you want to use AI and data analytics to sharpen decision-making?
Regardless of the goals, CIOs must ensure that AI adoption is purposeful, strategically aligned with business priorities/objectives, and backed by a solid implementation plan. By prioritizing business-driven outcomes, CIOs can ensure that generative AI seamlessly integrates into existing operations while delivering tangible, long-term value.
No 2: Access AI readiness beforehand
Prior to rolling ourt generative AI, CIOs must ensure that their organization is prepared for thise transition. Taking an AI readiness assessment should be an important consideration in this process, helping businesses evaluate their existing capabilities, identify gaps, and devise strategies for successful AI adoption.
Without fully understanding your organization’s preparedness, heading straight into generative AI deployment can lead to inefficiencies, failed implementations, or suboptimal AI performance. A readiness assessment ensures that:
- AI adoption aligns with business objectives.
- The organization has the necessary infrastructure and data quality.
- The workforce is equipped with the skills to leverage AI effectively.
Skipping this step will lead to wasted resources and underwhelming results. By assessing readiness upfront, CIOs can identify gaps and develop a clear roadmap, whether it’s improving data governance, investing in employee training, or upgrading IT infrastructure to ensure generative AI implementation is smooth and successful.
Become AI-ready: Download our AI preparedness guide for successful AI adoption
No 3: Address copyright, confidentiality, and compliance
Although generative AI is a game-changer, it also brings a legal and security concern that CIOs cannot afford to overlook. As AI-generated content becomes more prevalent, organizations face serious risks – from copyright infringement to data privacy breaches that could expose confidential information. Generative AI models, trained on vast amounts of data, may unintentionally generate content that mirrors copyrighted material, raising intellectual property concerns and potential legal disputes. Meanwhile, sensitive business data fed into AI systems could be leaked, misused, or even exploited by bad actors, posing major security threats.
To stay ahead of these challenges, CIOs must take a proactive stance. This means implementing strong data governance policies, ensuring AI models comply with copyright and privacy regulations like GDPR and CCPA, and securing AI-generated outputs from unintended risks.
Read more: Learn how to build trust and transparency with strong data governance in generative AI.
No 4: Focus on high impact use cases
Not every AI project delivers real business value. Therefore, CIOs and CTOs need to be strategic about where and how generative AI is applied. The key is to identify high impact generative AI use cases that solve pressing business challenges or unlock new opportunities.
To maximize AI’s value:
- Align AI with business needs – Focus on areas like customer experience, efficiency, or revenue growth.
- Cluster use cases strategically – Organize AI initiatives by domain (e.g., customer journey, finance) or function (e.g., virtual agents, content generation) for maximum impact.
- Think long-term – Some AI projects provide quick wins, while others require long-term investment. CIOs should balance both, ensuring early successes build momentum for broader AI adoption.
By targeting the right use cases, CIOs can ensure generative AI delivers tangible value and lasting business impact.
Book your consultation: Identify practical Gen AI use cases for your business with our POC
No 5: Ensure AI-ready architecture for scalable success
The effectiveness of generative AI is only as strong as the quality and accessibility of the data it relies on. Without a well-structured data architecture, organizations risk deploying generative AI models that generate irrelevant, inaccurate, or biased outputs. To unlock maximum business value, from cost reductions to improved decision-making, CIOs must ensure their AI models are properly integrated with internal, high-quality data sources.
To achieve this, CIOs should:
- Organize and standardize data
Structure both structured and unstructured data, ensuring improved traceability, consistency, and readiness for AI processing. Implement modern data pipelines to facilitate seamless data flow.
- Enable real-time data ingestion
Adopt scalable infrastructure capable of handling AI’s data-intensive demands, ensuring real-time ingestion from IoT devices, transactional systems, and external sources for up-to-date AI-driven insights.
- Leverage vector databases for contextual intelligence
Utilize vector databases to enhance generative AI’s ability to retrieve and generate contextually relevant responses, improving accuracy and reducing model hallucinations.
By investing in an AI-ready data architecture with scalable infrastructure, real-time data access, and advanced retrieval mechanisms, CIOs can enable faster, more accurate, and enterprise-grade generative AI solutions while maintaining robust data privacy, security, and governance standards.
No 6: Build a dedicated generative AI platform team
To scale generative AI effectively, CIOs must go beyond isolated AI experiments and integrate AI into the organization’s product and platform strategy. The best way to do this is by creating a centralized, cross-functional AI platform team that develops and maintains a standardized AI infrastructure for the entire enterprise.
A well-structured AI platform team ensures the deployment, monitoring, and governance of generative AI solutions. To build an effective team, CIOs must include specialized roles such as:
- Senior technical leader – Provides strategic direction and ensures AI initiatives align with business goals.
- Software engineer – Develops AI-powered applications and integrates models into existing systems.
- Data engineer – Designs and manages data pipelines, ensuring high-quality, AI-ready data.
- Machine Learning engineer – Builds, trains, and fine-tunes AI models for optimal performance.
- MLOps/AI Ops engineer – Focuses on model lifecycle management, monitoring AI performance, and ensuring reliability and compliance.
By investing in a strong AI platform team, CIOs can ensure that generative AI adoption is scalable, secure, and strategically aligned with business goals, turning AI from an isolated experiment into a true competitive advantage.
Integrate generative AI into your business with the right expertise
Generative AI presents a world of opportunities – but only for those who deploy it with foresight and strategy. The difference between success and failure lies in how well CIOs align AI with business goals, ensure data readiness, mitigate legal risks, and build scalable infrastructure. By mastering these six key insights, CIOs can turn generative AI from an experimental tool into a true driver of business transformation.
However, adopting AI at scale requires expertise and the right technology partner. Reach out to us at marketing@confiz.com to accelerate your generative AI adoption, build scalable solutions, and turn AI into a true competitive advantage.