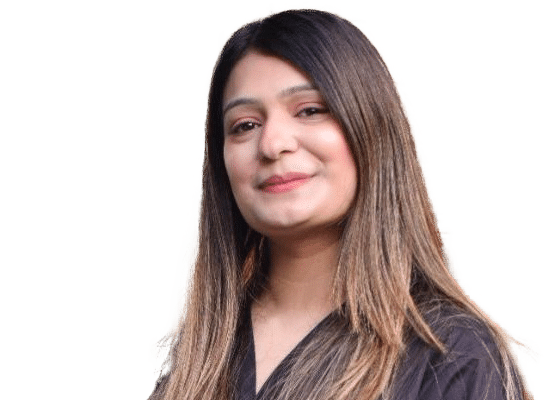
Data & AI Evangelist
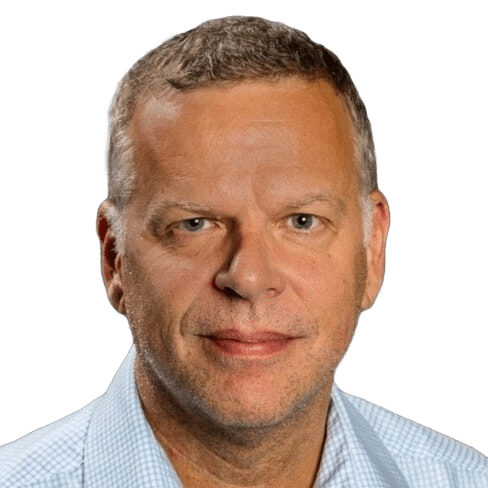
Principal Data Architect
Subscribe to the newsletter
All organizations generate data, but not all succeed at extracting its true value. A recent study indicates that many business leaders find their current approach to data analytics ineffective. 77% of these leaders believe that the dashboards and charts they receive do not always align with the decisions they need to make. Additionally, 72% think the available data only benefits data scientists or IT professionals.
72% of business leaders worldwide acknowledge that the sheer volume of data and lack of trust in the data hinders decision making. These findings highlight the pressing need for modern Business Intelligence and Data Analytics (BI) tools that enable business leaders and other employees in an organization to effectively harness data, provide actionable insights, and empower decision-makers.
One thing is certain: not all organizations need to immediately add BI tools into their tech stack. However, even having one effective tool can significantly simplify day-to-day work life, especially when making decisions based on data.
We understand that selecting the right BI tool for effective data storage, analysis, and prediction to enhance your business’s performance can be overwhelming, especially with so many options available. You’ve come to the right place for guidance.
This blog walks you through the basic concepts of data analytics and Business Intelligence (BI), followed by the right criteria for selecting the most suitable analytics and BI tools that align with your organization’s objectives.
Are both analytics and Business Intelligence the same?
When it comes to leveraging data and AI, analytics and Business Intelligence stand out as key approaches. Despite their mutual reliance on data, it is important to recognize that Business Intelligence and data analytics are two distinct concepts. Now, what is BI and analytics?
Business Intelligence (BI) refers to the processes, technologies, and tools used to transform raw data into meaningful insights for strategic decision-making within organizations. BI aims to enable businesses to make informed decisions by providing access to and analysis of relevant data.
On the other hand, data analytics focuses on revealing trends and patterns which in turn generate actionable insights to drive informed decision-making. With data analytics, organizations can refine their strategies, enhance operational efficiency, and adapt swiftly to changing market dynamics.
Here, we’ve highlighted two major differences between data analytics and business intelligence, which set both apart.
1. Distinguishing unstructured data from structured data
Data analytics operates with structured data but often begins with unstructured, real-time data. Data analysts are tasked with organizing and cleansing these data before storing them for future analysis.
Business Intelligence uses structured data to produce dashboards and reports. Structured data includes data stored in warehouses, tabulated databases, and other systems.
2. Utilization of insights versus generation of insights
Data analytics is primarily concerned with transforming and refining raw data into actionable insights, serving various purposes, including business intelligence.
However, the fundamental goal of Business intelligence is to facilitate decision-making by employing actionable insights derived from data analytics.
The importance of BI tools and how they support the modern data architecture
Every organization uses a business intelligence tool for a different purpose, ranging from improving strategy to execution. By consolidating data in a unified environment, these tools enable faster insights extraction and more efficient decision-making. Modern BI tools seamlessly integrate with your organization’s data sources, handling transport, storage, and transformation workflows, including machine learning and AI pipelines.
Prominent BI tools in the industry include Microsoft Power BI, Tableau, Sigma, and Qlik. Choosing the right BI tool is important, as it should not only meet technical requirements but also effectively support your company in achieving its business objectives.
Further readings: Explore how to use Power BI for Business Intelligence
BI and Analytics tool selection framework: Key factors to consider
At the foundational level, both Business Intelligence and analytics tools are indispensable for turning data into valuable insights. With countless considerations, choosing the right one seems quite overwhelming. However, we have focused our attention on four main categories that will simplify the decision. Let’s go through these in detail one by one.
1. Understanding the users and their needs
Making the right choice in selecting BI and analytics tools begins with the understanding of who will be using it and how. The first component of the framework entirely focuses on defining user personas and aligning their needs with the functionalities offered by a particular tool. Moreover, it also involves assessing the tool’s compatibility with back-end data platforms as well as users’ and developers’ learning curves.
Defining user personas
- Data analysts are the power users of analytics and BI tools who are tasked with conducting complex data analysis, creating dashboards, and generating reports. They require advanced functionalities like data mining, statistical modeling, and drill-down capabilities.
- Business users focus on utilizing and interpreting data to facilitate well-informed business decisions. They require user-friendly tools with drag-and-drop functionalities for report building, pre-built dashboards, and interactive data visualization.
Functionalities based on user personas
- Data analysts are responsible for data exploration, advanced analytics, data visualization, report building, and data storage.
- Business users are tasked with different responsibilities such as data integration, data management, data analysis, self-service analytics, and creating interactive visualization for sharing insights.
Learning curve for users
It’s important to consider the learning curve when evaluating how your team will use BI and advanced analytics tools. A complex tool will make users struggle with its functionalities and consequently miss out on critical insights that could drive strategic decisions. The steeper the learning curve of a BI and analytics tool, the more complex data-driven decision-making will be.
2. Considering your data platform
The second thing to consider is your data platform. This is to ensure that the chosen tool seamlessly integrates with your existing data infrastructure and provides access to the data you need for analysis. In a nutshell, choose a tool that describes your current data platform and whether it aligns with the modular, cloud, or decentralized framework (if you are considering moving it in the future).
Integration with existing data infrastructure
- Data Warehouses: BI tools often connect to data warehouses, which act as centralized repositories for structured data from various sources. Choose a BI tool that offers connectors or easy integration with your existing data warehouse.
Modular, cloud, decentralized framework
- Modular
This includes services for distinct data functions, such as data transportation, warehousing, and transformation. For instance, you can use Fivetran for data transport, Snowflake for data warehousing, and dbt for data transformation.
- Cloud
Platforms like Microsoft Fabric, Google BigQuery, or AWS Redshift often come with embedded business intelligence as an essential feature. Such platforms provide a comprehensive set of tools available within their ecosystem.
- Decentralized
Some organizations have separate databases instead of one, necessitating the need for a BI tool to unify the data being produced and gathered in separate environments. This tool needs to be able to take in and change data from many different sources, often without the support of a central data warehouse or Lakehouse. In such a scenario, it’s essential to carefully consider how well the BI tool you are considering can handle the amount of data stored in different locations.
Find out the key differences between data lake and data warehouse here.
3. Accounting for data transformation
When it comes to Business Intelligence and analytics, one of the main components that is often overlooked is accounting for data transformation. This means that data needs to be cleaned, manipulated, and transformed into a usable format before it is analyzed to extract meaningful insights. However, to make the right choice for a BI tool, determine a BI tool that has built-in features for cleansing and transformation. The goal is to make the most of tools in a way that fits your architecture, ensuring a streamlined data flow and efficient utilization of system resources.
When evaluating BI tools based on their data transformation capabilities, keeping note of the following factors will help you make the right choice:
- Ease of use
- Flexibility and customization
- Scalability and performance
- Integration with external data integration approach, ETL (Extract, Transform, and Load)
If you want to ensure that your BI tool is contributing to efficient data management, maximize your data platform’s computational power for data transformation while maintaining centralized data logic and dimensional models. This enhances efficiency and streamlines future migrations. If you’re already using a BI tool for complex data, transition to your cloud platform. This ensures a unified, reliable source of truth for your data, making management and system adaptation easier in the future.
4. Migration to BI or analytics tool
Transitioning from an existing system to a new BI tool necessitates a well-defined migration plan to ensure a smooth and successful switch. Make sure that no data is lost or corrupted during the transition. This includes both extraction, transformation, and loading (ETL) and extraction, loading, and transformation (ELT) to ensure data integrity during this phase. Before making the transition,
- Effectively train your users on the new BI tool functionalities and interface. This minimizes resistance to change and ensures users can leverage the new tool’s full potential.
- Thoroughly test the new BI tool functionality and data migration before full deployment. This ensures a smooth transition and minimizes potential issues.
- Assess how heavily users rely on the existing tool and plan training accordingly.
Summing up
Business success has always depended on critical decision-making. The difference today is that the organizations are generating a huge amount of structured and unstructured data. Leveraging this data effectively requires the right tools. By following a structured BI and analytics framework for tool selection outlined above, you can choose the right data analytics and BI tools that empower your organization to make data-driven decisions, gain a competitive edge, and achieve long-term success.
Struggling with a large pool of unstructured and low-quality data and failing to leverage insights for data-driven decision-making? Get in touch with us. Our data analysts, data engineers and data scientists will understand your challenges and transform your operations with the power of business intelligence. Leveraging our expertise in data and AI and through our expertise in Business Intelligence, we can drive a data-driven revolution for your business. For more information, contact us at marketing@confiz.com.