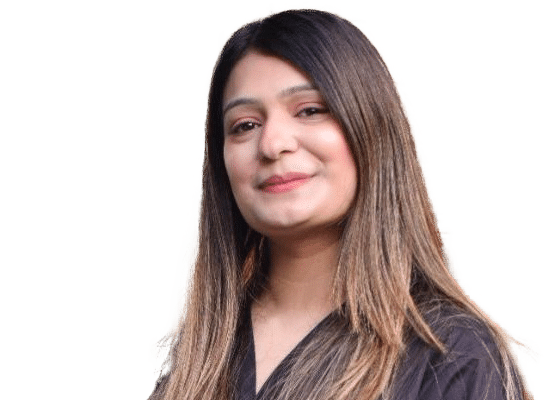
Data & AI Evangelist
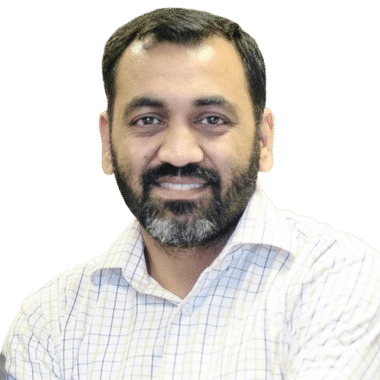
Director of Analytics and Business Intelligence
Subscribe to the newsletter
Working with data in the cloud has become a new normal for modern businesses. The flexibility, scalability, and accessibility it offers to access data anytime, anywhere has made it a must-have for data-driven businesses. With virtualized access to data through cloud data platforms, businesses are now processing and gaining insights from their data to drive transformation and value creation.
Since organizations are now emphasizing modernizing their data systems to accelerate impact and capitalize on business opportunities faster, cloud data platforms are proving to be highly effective in fulfilling these goals. These platforms offer a robust suite of tools and services to manage the entire data lifecycle, from data ingestion and storage to data analysis and visualization. This holistic approach ensures businesses efficiently handle their data, turning raw data into actionable insights that propel growth and innovation.
However, the intrigue lies in the fact that two leading contenders, Databricks and Snowflake, have emerged as the go-to platforms for data-backed businesses managing their data workloads on the cloud. Both platforms offer distinct advantages and cater to different aspects of data management and analytics, sparking curiosity and a desire to learn more about their unique features.
This blog will provide a high-level overview of the detailed differences between Databricks and Snowflake, highlighting their features to help you make the right choice for your cloud data warehousing needs.
To begin a meaningful comparison, let’s start with understanding the basics of cloud data platforms.
What are cloud data platforms?
Traditional data warehouses are giving way to cloud data platforms. These cloud-based data platforms manage and store data workloads in the cloud, offering democratized access that breaks down internal silos. Business users across the organization can now access and analyze both structured and unstructured data in the cloud with ease. This flexible solution empowers businesses with on-the-go analytics, leading to faster and more efficient decision-making.
However, as the data workload keeps fluctuating and unstructured data volume keeps multiplying (which now accounts for 80-90% of all the generated data), cloud data platforms are increasingly gaining traction for its:
- Ability to scale storage without the need for physical hardware.
- Support for diverse data types and formats (structured, semi-structured, and unstructured data).
- Rapid access to data from anywhere, both remotely and on-prem.
- Built-in security measures to protect data integrity and privacy.
- Pay-as-you-go pricing model that optimizes costs based on actual usage without investing in expensive hardware and infrastructure.
The data Lakehouse pioneer: Databricks
Databricks stands out as a cloud-based data analytics platform designed for large-scale data processing and machine learning tasks. The foundation of Databricks is built on Apache Spark that provides an integrated environment to data scientists, data analysts, and data engineers to collaborate seamlessly on data-driven projects. On top of that, Databricks combines the benefits of Delta Lake, ML flow, Apache Spark, and data warehouse to simplify end-to-end data analytics process.
This unified data analytics platform is provided as a managed service, streamlining the workflow for data engineering and data science. With features like collaborative notebooks and integrated libraries for machine learning, Databricks offers a comprehensive workspace for data professionals. Its scalable architecture ensures efficient data processing and model training, making it a top choice for organizations aiming to leverage big data and AI.
A cloud-native data warehouse: Snowflake
Databricks has established itself as a powerful force in the data analytics landscape, but let’s shift gears and explore another key player: Snowflake.
Snowflake is a cloud-native data warehouse built from the ground up for the modern data environment. Unlike Databricks, which operates as a unified data platform encompassing various functionalities, Snowflake focuses on a specific, critical task, i.e., data warehousing. Snowflake data warehousing has a decoupled storage and compute architecture. This means it allows independent scaling of data workloads, enabling users to optimize performance and cost by adjusting storage and compute resources separately based on their needs.
While not a one-stop shop for the entire data lifecycle like Databricks, Snowflake excels at data warehousing in the cloud. Its focus on scalability, performance, and security makes it a compelling choice for organizations seeking a reliable solution for storing and analyzing vast amounts of data.
Databricks vs Snowflake: A detailed comparative analysis
Over time, Snowflake and Databricks have earned their reputations as the leading all-in-one cloud data platform solutions, providing businesses with robust capabilities for managing and analyzing vast amounts of data. Both these platforms cater to distinct needs and offer unique advantages to their users. While they may not be a one-size-fits-all solution, understanding the differences between them is indispensable for choosing the perfect platform to propel your data-driven journey.
Let’s know the differences between Databricks vs Snowflake and uncover what features actually make them good enough to work with your ever-evolving data.
Difference 1: Databricks vs Snowflake – Architecture
Databricks and Snowflake have distinct architectural designs that cater to different data processing needs. Databricks is built on Apache Spark and follows a unified architecture that integrates data engineering, data science, and machine learning into a single platform. This architecture allows for seamless collaboration between data scientists and engineers, providing an environment where they can work together on complex data workflows.
On the other hand, Snowflake data warehouse has a shared data architecture that separates storage and compute resources. This decoupled design enables independent scaling of storage and compute, allowing organizations to optimize performance and costs more effectively.
Difference 2: Databricks vs Snowflake – Service model
Databricks is a PaaS offering that provides a comprehensive environment for developing, deploying, and managing data analytics and machine learning applications.
Snowflake’s SaaS model provides a streamlined, fully managed data warehousing solution that prioritizes ease of use and efficiency. It caters to a broader range of users, including those with limited technical expertise.
Difference 3: Databricks vs Snowflake – Data structures
When it comes to data types, Databricks and Snowflake offer different capabilities. Databricks excels in handling various data types, including structured, semi-structured, and unstructured data. Its foundation on Apache Spark enables efficient processing of large datasets, making it suitable for diverse data workloads, such as streaming data and big data analytics.
Snowflake data platform also supports multiple data types, but its strength lies in structured and semi-structured data. Snowflake’s architecture is optimized for complex SQL queries, making it a powerful tool for data warehousing and analytics.
Difference 4: Databricks vs Snowflake – Ease of use
Ease of use is a crucial factor when choosing between Databricks and Snowflake. Databricks provides an interactive workspace with collaborative notebooks, which can appeal to data scientists and engineers who prefer a code-centric environment.
Snowflake is designed with simplicity in mind, offering a user-friendly interface. This platform focuses on ease of use and makes it accessible to a broader range of users, including business analysts and data professionals who don’t have extensive coding background.
Difference 5: Databricks vs Snowflake – Storage
The Databricks Lakehouse platform has a Delta Lake, an open-source storage layer that allows data to be processed in the lake instead of being loaded into the data warehouse.
Snowflake, on the other hand, stores structured data in a proprietary format for quick data querying and transformation. Snowflake’s data cloning feature allows users to create separate objects from existing data without requiring actual replication. This not only reduces storage costs but also provides control over data access and governance. As long as both the original and cloned data remain unaltered, storage optimization is ensured.
Snowflake also supports data shares, allowing users to create shared access points across different users and applications. These shares serve as abstract connections, facilitating controlled data flow in and out of the Snowflake environment. This enables organizations to enforce specific quality and governance policies on each data flow. It’s important to note that Snowflake incurs costs on data reads, meaning that accessing data generates costs, which applies to both data cloning and data sharing.
Both Snowflake and Databricks are cloud agnostic and run efficiently on major cloud service providers, such as Microsoft Azure, Google Cloud Platform, and Amazon AWS.
Difference 6: Databricks vs Snowflake – Target market
Databricks and Snowflake cater to different target audiences. Databricks is designed with a focus on data engineers, data scientists, and analysts who require a unified platform for complex data processing, analytics, and machine learning tasks.
Snowflake is tailored for business analysts, data professionals, and decision-makers who need a user-friendly, scalable, and secure data warehousing solution. Its SaaS model prioritizes ease of use, making it accessible to a broader range of users.
Besides Snowflake, Databricks has also been in a leading debate with Microsoft Fabric, as both these platforms serve similar purposes within the analytics landscape.
Read more: Understanding the differences between Databricks and Microsoft Fabric.
Difference 7: Databricks vs Snowflake: Cost comparison
When choosing between Databricks and Snowflake, it’s important to consider how each platform’s pricing model fits your needs. Databricks charges based on the compute and storage resources you use, so costs can vary with the intensity of your workloads.
Snowflake, on the other hand, offers a pay-as-you-go model where you pay for the compute time and storage separately. Understanding these differences can help you better manage your budget and choose the platform that best aligns with your data processing needs.
Which platform to choose or ditch?
When migrating from a legacy system to modern data architecture, Snowflake is often preferred if your environment leans towards traditional databases. Its familiar SQL-based interface and ease of integration with existing systems make it a smooth transition for data warehousing and reporting.
On the other hand, Databricks is ideal for those adopting a data lake or Lakehouse architecture. It supports large-scale data processing and machine learning workflows, making it a robust choice for advanced analytics, though it may require a steeper learning curve.
Read more: A quick comparison of data lake vs data warehouse vs data Lakehouse.
Choosing between the modern cloud data platforms: Databricks vs Snowflake
As both cloud data solutions are helping modern businesses to streamline their data analytics, the debate between choosing the two leading cloud data platforms often boils down to finding the best fit for your specific needs. The following are some of the factors that will help you simplify your decision-making process for the right platform. These include:
Workload requirements
If your organization deals with complex data processing, machine learning, and real-time analytics, then Databricks is the go-to option. While Snowflake is best suited for business intelligence and data warehousing needs.
Ease of use
If your organization is looking for a user-friendly interface and SQL-based querying, Snowflake is a strong choice. However, Databricks require more technical expertise to manage and operate.
Budget-friendly
While Snowflake’s upfront costs are higher, their pricing structure is clearer and easier to predict. Databricks, on the other hand, offers a pay-per-use model that can be more complex to estimate.
Technical expertise
Snowflake’s intuitive interface makes it an ideal option for teams without extensive data engineering or machine learning expertise. Databricks, while more flexible and powerful for experienced users, has a steeper learning curve.
Closing thoughts
Both Databricks and Snowflake have proven to be formidable contenders, each offering unique strengths tailored to different business needs. Choosing between Databricks and Snowflake ultimately depends on your organization’s specific requirements, workload characteristics, and strategic goals.
Whether you need the powerful data processing capabilities of Databricks or the streamlined data warehousing experience of Snowflake, both platforms offer robust solutions to help you unlock the value of your data. At Confiz, we specialize in providing Databricks consulting services to help you make the most out of this advanced cloud data platform. Reach out to us at marketing@confiz.com and explore how we can help you achieve your data-driven goals for your organization.