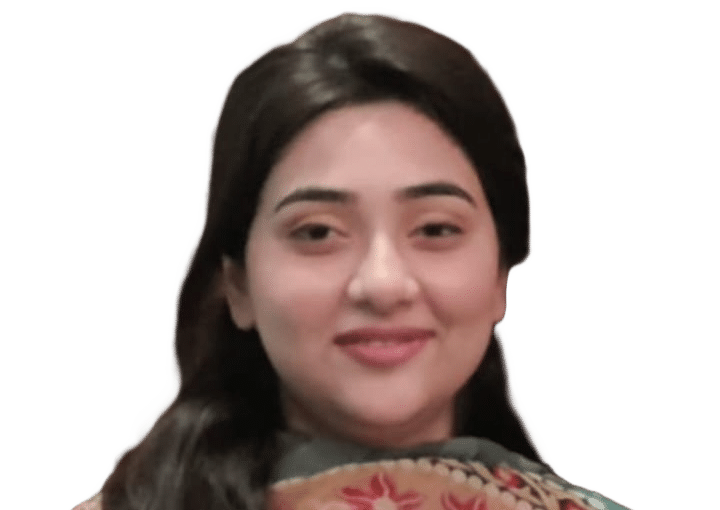
Global Content Lead - Tech & Consulting
Subscribe to the newsletter
Big data is rapidly becoming a driving force behind the global economy. At present, a whopping 2.5 quintillion bytes of data is being produced daily and the sheer volume is still expected to grow. As a result, organizations are faced with the challenge of effectively managing and leveraging the vast and diverse pool of data generated daily.
Two prominent solutions to this challenge are Data Lake and Data Warehouse. Widely used by enterprises, these solutions serve as indispensable tools for data management, data integration, data storage and data analytics.
The data lake vs. data warehouse comparison is an ongoing debate among data analysts. While both data lake and data warehouse serve as data storage and analysis repositories, they diverge considerably in their architecture, approach, and functionalities.
This blog will analyze the difference between a data warehouse and a data lake and explore how they contribute to effective data management.
What is a data lake?
A data lake is a centralized repository that stores vast amounts of raw data in its native form. It allows organizations to store diverse data types, such as structured, semi-structured, and unstructured data, without predefining its structure or schema.
Data lakes architecture leverage distributed processing and storage technologies, enabling advanced analytics, machine learning, and business intelligence on massive datasets. Examples of data lake technologies include Apache Hadoop, Amazon S3, Google Cloud Storage, and Microsoft Azure Data Lake Storage.
Data lakes are vital to modern data-driven organizations, providing a flexible solution for data storage, integration, and analysis. However, ensuring data governance, quality, and access controls remains essential to maximize their benefits.
What is a data warehouse?
A data warehouse is an optimized repository that consolidates data from various sources within an organization. It supports business intelligence (BI) and analytical activities by providing a unified data view.
Data warehouses are subject-oriented, time-variant, and non-volatile, meaning they focus on specific business areas, capture historical data changes, and preserve data integrity.
Data is transformed, cleaned, and organized in a data warehouse, making it suitable for complex queries and analysis. Data warehouse architecture involves a systematic approach to organizing data from various sources, transforming it into a consistent format, and making it available for analytical queries and business intelligence. Examples of data warehouse technologies include Amazon Redshift, Google BigQuery, Microsoft Azure Synapse Analytics, and Snowflake.
Building a data warehouse empower businesses to make data-driven decisions, facilitating data analysis, reporting, and performance tracking across various departments and functions.
Data lake versus data warehouse – An overview
Understanding the difference between data lake and data warehouse is necessary for organizations seeking to optimize their data management and analytics strategies. Both data repositories offer unique advantages and cater to specific data requirements. Some of the key differences between data warehouse and data lake are:
1) Data structure
Data lakes store data in its raw and native format without a predefined schema. This allows for ingesting diverse data types, including structured, semi-structured, and unstructured data. The flexibility in data structure makes data lakes suitable for accommodating data from various sources without requiring extensive data transformation.
Data warehouses, in contrast, enforce a predefined schema before storing data. They follow a structured approach, organizing data into tables with fixed schemas. This ensures data consistency and facilitates optimized query performance for reporting and analysis.
2) Data processing approach
Data lakes adopt a “schema-on-read” approach. Data is transformed and structured during the data retrieval process, allowing data scientists and analysts to apply different schemas and interpretations based on specific analysis requirements. This flexibility empowers users to explore and analyze data without the constraint of a predefined schema.
Data warehouses use a “schema-on-write” approach. Data is transformed and structured before being loaded into the warehouse, ensuring data consistency. This approach reduces processing overhead during data retrieval and provides a stable BI reporting and analytics environment.
3) Data integration
Another key difference between data lake and data warehouse is in terms of data integration. Data lakes are designed to accommodate data from various sources without extensive data transformation. They support real-time and batch data ingestion, making them ideal for handling diverse data streams, IoT devices, social media, and log data. Effective data lake integration ensures that all these varied data sources can be seamlessly brought together, enabling comprehensive analysis and insights.
Data warehouses require data to be pre-integrated and transformed before loading. Data integration involves cleansing, aggregating, and aligning data from different sources. This upfront data preparation ensures data quality and consistency for analytical purposes.
4) Data storage and scalability
Data lake storage is built on distributed storage systems, such as Hadoop Distributed File System (HDFS), or cloud-based object storage like Amazon S3 or Azure Data Lake Storage. This architecture enables data lakes to scale horizontally, easily accommodating large volumes of data.
Data warehouses utilize relational database management systems (RDBMS) or columnar databases for structured storage. Although they offer efficient data retrieval for analytical queries, traditional data warehouses may need help dealing with the scalability of massive data volumes.
5) Analytical capabilities
Data lakes offer exploratory and ad-hoc analytical capabilities. With the flexibility of “schema-on-read,” data scientists can perform advanced analytics, machine learning, and data discovery, making data lakes a playground for data exploration. Moreover, the integration of Data Lake analytics further enhances these capabilities and allows more efficient and complex data analysis to data professionals.
Data warehouses excel in predefined and structured analytical operations. They are optimized for running complex queries and providing consistent and accurate reporting, making them well-suited for business intelligence and operational analytics.
6) Data governance and security
Data lakes often face data governance and security challenges due to their schema-less nature. Ensuring data quality, access controls, and data lineage is important for maintaining data integrity without a predefined schema. Implementing effective data lake governance address these issues by establishing policies and procedures that oversee the management and use of data within the lake. Moreover, it also ensures that data remains secure, high-quality, and properly tracked.
Data warehouses are designed with built-in data governance and security features. Data warehouse governance provide role-based access controls, data encryption, and auditing capabilities, making them more suitable for compliance and regulatory requirements.
Conclusion
Data lake and data warehouse are both essential elements of a comprehensive data management and analytics ecosystem. Data Lake stores diverse data types without predefining its structure, while Data Warehouse consolidates data from various sources with predefined schemas.
Each solution offers unique advantages and caters to specific data requirements. When used harmoniously, they provide a powerful and flexible approach to data management. Organizations can make informed decisions to enhance their data optimization strategies by understanding the difference between data lake and data warehouse.
Ready to benefit from advanced data management services? Contact us at marketing@confiz.com and let our data experts walk you through the benefits and help you choose the best option for your organization.